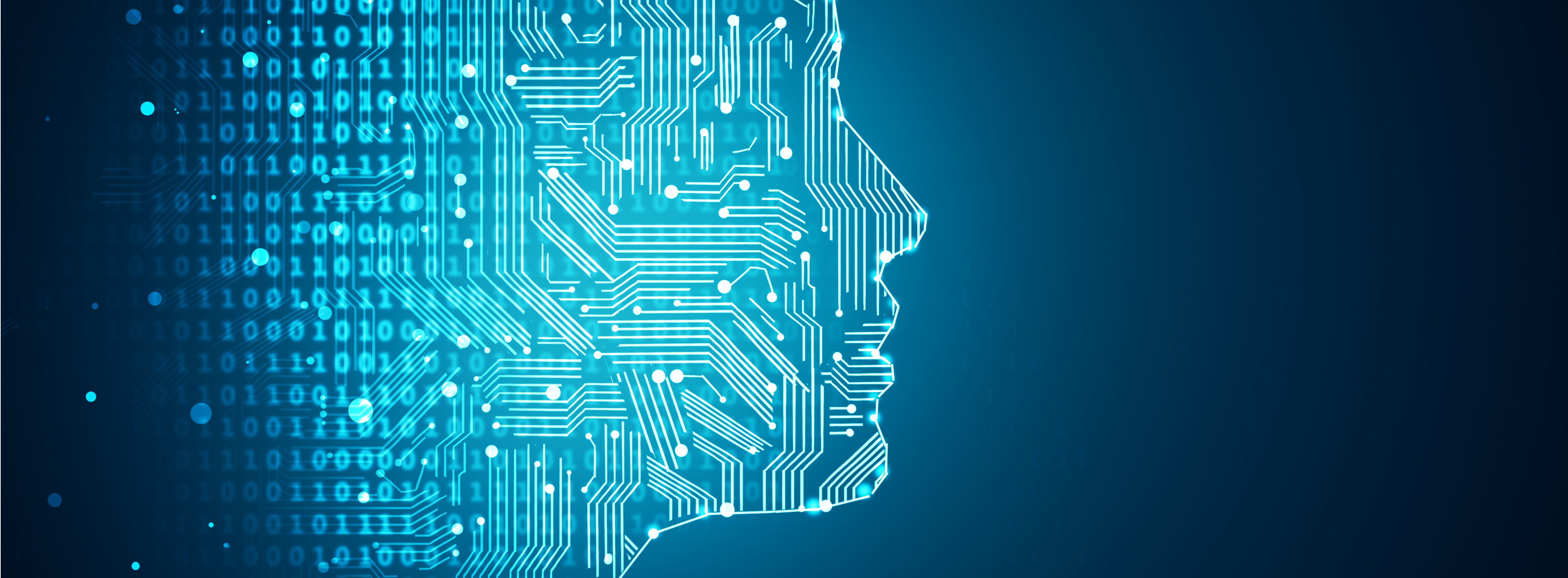
Artificial intelligence and machine learning in genomics have become something of buzzwords over the last few years. Genomics itself has been at the forefront of the information revolution that is sweeping across healthcare. Now, the debate is shifting towards how complex computing can enable clinicians, doctors and scientists to truly capitalise on the data goldmine that genomics is, and this is where terms such as ‘AI’ and ‘machine learning’ come in.
The first thing to address is the terminology. Artificial intelligence and machine learning are two of the hottest buzzwords around this field at the moment and, though they mean quite different things, they are often used interchangeably.
Artificial intelligence is not as dramatic as Hollywood portrays. In many ways it has already become something we take for granted; Google Translate, facial recognition or voice operated devices such as Alexa or Siri. These are systems programmed to mimic or complement human thinking. Although they cannot understand nuance, they are flexible programmes capable of solving a wide range of retrieval questions, essentially locating and connecting you with the information for which you are looking. For example: ‘Siri, what’s the best use for artificial intelligence?’
Machine learning on the other hand is giving a computer a mass of data and allowing it to generate and alter its own algorithms to come up with solutions or patterns. It can design and modify itself – learning for lack of a better word – without being explicitly programmed.
Breaking Down the Data
The traditional approach to solving problems with AI is to give the computer a set of rules to work within, a task to achieve, and then apply brute computing force to the problem, like trying to crack a pin number by trying every possible combination.
With machine learning we give the computer the data and a goal and it defines its own rules and algorithms to reach a result. For example, a wealth of data about people who have suffered heart attacks is fed into the system and its algorithms quickly work through the data, so, when it has seen a million patients it is able to make predictions about groups or individuals who may be at various levels of risk of heart attack. It can’t however ask why any ‘why’ or ‘how’ questions and its answers will always be numerical and statistical in nature.
Computing as it is commonly used operates by a user giving a specific instruction and the machine returning a specific bit of data, for example, what was the average age of our heart attack patients? Here the computer would give a single answer and finish. With machine learning it would be simultaneously asking that and more questions; where did they live? Did they smoke? Was this their first medical contact? It can then cross reference the data. It is from these steps where its advantage comes.
The Limitations of Technology
However, therein lies the great strength and weakness of machine learning. It is excellent at tasks such as pattern recognition or crunching huge amounts of data to reduce it down to the critical decision points. However, it can’t second guess itself and it can’t choose to ‘think’ differently. Machine learning is tied to algorithms; it can only speak in maths and can’t ask deeper, expansive questions, and it can only be as good as the data it is given.
Machine learning is nothing more than a statistic driven technology, but it is a very fast one, capable of carrying out a wide range of complex processes by learning from their inputs rather than reading from sets of pre-programmed rules.
That line between machine learning and statistics is blurry at best with some experts preferring the name ‘statistical learning’. The field itself grew out of the artificial intelligence community of the late 90’s and has mostly focused on the analysis of large, heterogeneous data sets.
Artificial Intelligence & Machine Learning in Genomics & The World
It is estimated that 90% of all the world’s digital data is less than five years old. Across genomics people are talking about the vast amount of information currently being generated through sequencing; machine learning could be the key to transforming this from a daunting burden into a data goldmine.
Machine learning methods have already been applied to a number of problems faced in genomics such as annotating genomic sequence elements, identifying splice sites, promoters, enhancers and positioned nucleosomes. Sapientia, for example, uses Exomiser, a tool that uses algorithms to annotate and prioritise variants from whole-exome sequencing to assist in the diagnosis of rare disease.
“Sapientia puts more information in front of the user than any other platform. Machine learning can’t take clinicians all the way to fully automated diagnosis because you always need the applications of human experience, for example, corner cases. The ‘state of the art’ for this is the empowerment of the experts by organising that data into one place in an easily comprehensible fashion. That is essentially how we got diagnosis times down from 5 years to 5 days. All forms of AI or machine learning are about multiplying human effort rather than replacing it.” Said Alan Martin, Head of Innovation at Congenica.
The promise of machine learning for genomics is enormous if it could be fulfilled. It could mean near perfect diagnoses, optimised medication and treatment choices, accurately predicted readmissions, identified high risk patients and general empowerment of the personalisation of medicine whilst all the time seeing costs minimised.
The biggest machine learning projects in the world are still in their early stages, especially those that look to eventually use real artificial intelligence. The biggest and best funded of these is London-based Google DeepMind Health. The Alphabet backed technology is currently working with Moorfields Eye Hospital in developing methods to tackle macular degeneration in aging eyes and with University College London Hospitals to detect differences in healthy and cancerous tissues and aiding accuracy in radiation therapies.
Machine learning will undoubtedly become a key appliance in a genetic clinician’s toolbox but it will be limited in scope, best used in niche and specialised areas where specific questions can be answered, or in studies that have a large data set. These situations offer the optimal set-up, with questions and data specific enough to create actionable interpretations. In comparison to feeding a computer information on patients that have suffered heart attacks, it would be akin to giving a computer data on all deaths in the last year and asking, ‘what happened?’
Legal ramifications may also affect adoption of machine learning, in particular for the healthcare industry in which the use of ‘black box’ style algorithms (where inputs go into the programme and outputs are produced with little explanation of how it ventured from point A to point B) may not be acceptable as the law demands increasing transparency.
Sebastian Thrun, former director of the Artificial Intelligence lab at Stanford University, said: “AI and ML are about magnifying human ability. The industrial revolution amplified the power of human muscle. When you use a phone you amplify the human voice. You cannot shout from New York to California. This cognitive revolution will allow computers to amplify the capacity of the human mind in the same manner.”